In today’s data-driven landscape, businesses grapple with vast amounts of information, especially in due diligence processes. This article explores the pivotal role of artificial intelligence (AI) in reshaping due diligence, with a specific focus on enhancing financial fraud detection and risk management, unravelling its implications and advantages. According to Juniper Research, global spending on AI-driven financial fraud detection and prevention platforms is projected to exceed $10 billion by 2027, indicating a significant surge from USD 6.5 billion in 2022. This surge is attributed to the escalating sophistication of fraudulent activities. Employing advanced AI techniques, merchants and issuers aim to combat these crimes by identifying fraudulent payment trends on a large scale
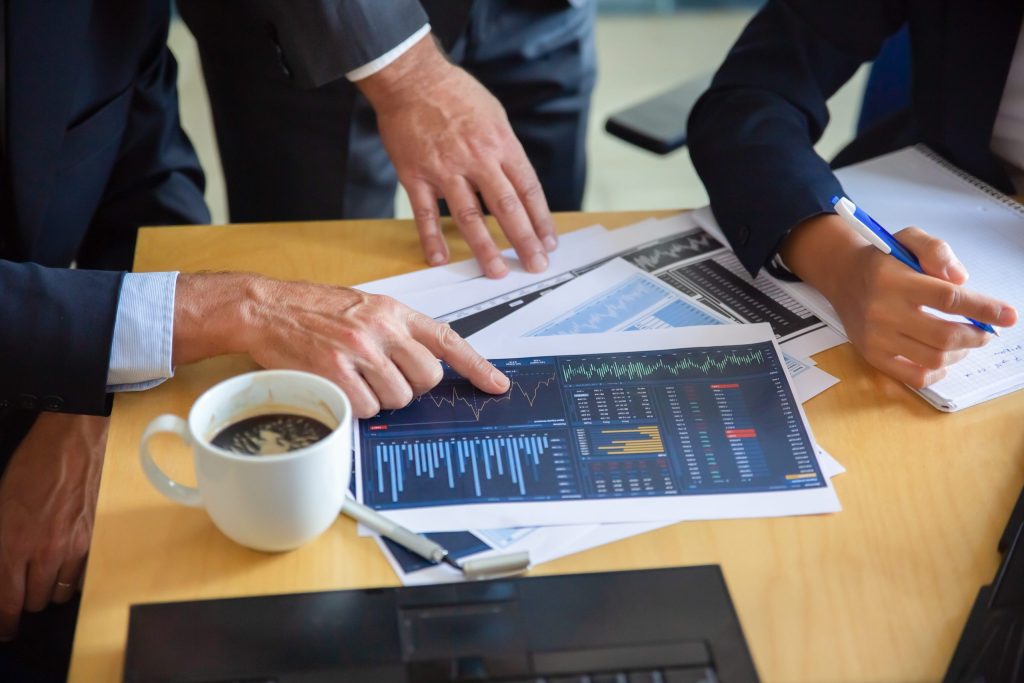
Understanding Due Diligence
Due diligence is a critical investigation, audit, or review conducted to validate facts or details pertaining to a matter under consideration. In the financial world, due diligence involves a meticulous examination of financial records before engaging in any proposed transaction.
Challenges in Traditional Due Diligence
Traditional due diligence methods are characterized by manual processes, which often present several challenges:
Time Constraints:
Thorough due diligence can be time-consuming, leading to delays in decision-making and missed opportunities.
Data Overload:
The vast volume of data to be analyzed can overwhelm human analysts, hindering the identification of crucial patterns or anomalies.
Subjectivity:
Human judgment introduces biases, resulting in inconsistent interpretations and conclusions.
Why AI is Redefining Due Diligence
The traditional method of reviewing numerous contracts and documents manually is time-consuming, expensive, and prone to inaccuracies. AI-driven due diligence streamlines processes, enhances accuracy, and reduces the risk of bad investments.
The Transformative Role of AI Due Diligence
The emergence of AI has ushered in a paradigm shift in due diligence processes. Here’s how AI is reshaping the landscape:
Data Aggregation and Analysis:
AI facilitates swift collection, organization, and analysis of structured and unstructured data, providing a comprehensive view of a company's health.
Pattern Recognition:
Machine learning algorithms uncover hidden correlations and anomalies within data, enhancing the depth and accuracy of due diligence.
Automation of Repetitive Tasks:
Mundane tasks such as data entry and cross-referencing are automated, allowing human analysts to focus on strategic evaluation.
Risk Assessment:
AI algorithms evaluate various risk factors associated with a business, enabling stakeholders to make informed decisions based on comprehensive risk profiles.
Document Review:
AI-powered tools streamline document-heavy aspects of due diligence by automatically extracting relevant information from documents.
Background Checks:
AI conducts comprehensive background checks on individuals and companies, identifying potential risks and ensuring regulatory compliance.
Financial Analysis:
AI analyzes financial statements and market trends, providing valuable insights for assessing a company's financial health.
Transaction Monitoring:
AI monitors transactions in real-time, flagging suspicious activities and enhancing risk management.
AI-Enabled Solutions and Use Cases for Due Diligence, Financial Fraud Detection and Risk Management
As financial institutions increasingly recognize the pivotal role of technology in combating financial crimes, a shift in budgets towards AI and machine learning solutions becomes evident. This transition signifies a departure from traditional rules-based systems towards AI-driven approaches, essential for scaling FinCrime programs effectively.
Financial institutions are exploring various AI applications to streamline their compliance processes. One prevalent use case involves leveraging AI to auto-adjudicate false positive alerts generated by transaction monitoring systems, name screening, and adverse media screening. By harnessing machine learning models as the initial line of defense, banks can significantly reduce the burden on human analysts and enhance operational efficiency.
AI-Powered KYC (Know Your Customer) And Customer Due Diligence (CDD)
Know Your Customer (KYC) stands as a pivotal mechanism in the financial sector, aimed at combatting fraud and upholding the integrity of financial transactions by enabling institutions to authenticate customer identities, assess risk profiles, and identify suspicious activities. Regulatory compliance intertwines with the integration of Artificial Intelligence (AI), marking a significant trend as institutions navigate intricate financial regulations. AI and Machine Learning (ML) technologies streamline KYC processes by automating identity verification, enhancing risk assessment, and bolstering fraud detection, ensuring accuracy and efficiency while reducing operational costs. The benefits of AI for fraud detection and Anti-Money Laundering (AML) compliance are notable, with studies indicating potential time savings of up to 60-70% in due diligence processes. Real-world examples highlight the practical applications of AI in the financial sector, reinforcing its role in ensuring compliance. Looking forward, regulatory authorities will play a vital role in shaping the adoption of AI and ML in KYC and AML compliance, with projected widespread adoption by 2027, underscoring the importance of these technologies in maintaining financial integrity. Best practices in KYC compliance emphasize leveraging technology, comprehensive procedures, and ongoing diligence to maximize protection against fraud, while fostering collaboration among banks, regulatory bodies, and technology providers to streamline processes and enhance fraud prevention capabilities across the sector. AI can analyze and verify customer identity documents like passports and utility bills in real-time, reducing manual review time and improving accuracy. Report by Deloitte emphasizes the necessity of harnessing analytics and technological innovations to enhance KYC processes swiftly. It underscores the urgency for financial institutions to adopt advanced technologies like AI and machine learning to streamline data collection and adapt to changes efficiently, ultimately improving operational effectiveness.
Leveraging AI to Combat Money Laundering and Other Financial Crimes
Financial fraud and compliance are reaching a critical point as criminals get more sophisticated, prompting financial institutions to make use of emerging technologies. Traditional Anti-Money Laundering (AML) systems are inadequate, with high false-positive rates, leading many institutions to adopt AI-driven solutions. AI detects money laundering through specialized algorithms analyzing vast data pools for suspicious activity, predicting future user behavior, enhancing due diligence processes, and automating suspicious activity reports, streamlining compliance efforts. Its ability to manage unstructured data further aids AML compliance by facilitating transaction monitoring and sanctions screening. Despite the rise in financial crimes, with 56% of organizations experiencing fraud in the past two years, according to PwC's Global Economic Survey in the UK, many institutions have been slow to fully adopt AI in fighting financial crime, remaining dependent on outdated systems. However, early adopters have embraced AI platforms to detect financial crimes faster and more effectively, enabling real-time responses to suspicious activities and staying ahead of criminals.
AI and Machine Learning for Credit Decisions
Artificial Intelligence (AI) revolutionizes credit decisions in banking apps development, offering faster, more accurate assessments of potential borrowers at reduced costs. Utilizing alternative credit scoring, AI employs sophisticated rules to differentiate between high default risk applicants and credit-worthy individuals lacking extensive credit histories. Moreover, AI ensures objectivity, eliminating human biases critical in financial app development. Digital banks and loan-issuing apps leverage machine learning algorithms to evaluate loan eligibility and provide personalized options. For instance, U.S. automobile lending companies report a 23% annual reduction in losses after implementing AI.
Big Data Analytics for Risk Management
AI plays a pivotal role in risk management within financial services, boasting immense processing power to handle vast data sets swiftly. Cognitive computing aids in managing structured and unstructured data, facilitating early identification of potential future issues by analyzing historical risk cases. AI can analyze vast amounts of customer data to assess creditworthiness more accurately. This allows banks to make informed lending decisions and minimize the risk of defaults. Studies by McKinsey show AI-powered credit scoring can improve loan default prediction accuracy by up to 15% .
Transaction Monitoring: Enhancing Efficiency and Accuracy
Transaction monitoring systems often inundate banks with a high volume of alerts, leading to manual reviews and resource-intensive processes. AI solutions offer insights into client transactions by integrating data from disparate sources, thereby reducing false positives and enabling quicker decision-making. Furthermore, machine learning models trained on human analyst decisions can automate and improve the accuracy of alert reviews, optimizing resources and enhancing risk management.
Addressing Challenges in Name and Adverse Media Screening
In name screening and adverse media screening, financial institutions face challenges related to data volume and accuracy. AI-driven solutions provide elastic scalability to handle spikes in volume and alleviate backlogs. By automating the review process and incorporating entity recognition and sentiment analysis, AI streamlines operations, improves accuracy, and reduces manual effort.
Fintechs Fighting Fraud with AI: Real-World Examples
Barclays:
The Financial Conduct Authority (FCA actively encourages innovation in RegTech and supports initiatives that leverage AI for fraud detection. For instance, Barclays Bank uses AI to analyze customer behavior and flag suspicious transactions in real-time, significantly reducing fraud losses
ICICI:
The Reserve Bank of India (RBI) has issued guidelines promoting the use of AI for fraud risk management. Many Indian banks, including ICICI Bank, are adopting AI-powered solutions to analyze vast datasets and identify anomalies that might indicate fraudulent activity.
JP Morgan:
The company utilizes AI for various purposes, including fraud detection, risk management, customer service automation, and investment analysis. They leverage advanced algorithms and machine learning models to enhance efficiency and decision-making across their financial services operations.
Capital One:
Capital One employs machine learning to bolster Anti-Money Laundering (AML) efforts, swiftly adapting to evolving criminal behaviors and prioritizing alerts based on risk levels. This collaboration between machine learning experts and AML professionals ensures greater efficiency and accuracy in detecting suspicious activities, enhancing financial security measures.
Bank of America:
They utilize AI-powered Erica, a virtual assistant that helps customers manage their finances. Erica can also detect and alert users about potentially fraudulent activity on their accounts.
PayPal:
This online payment platform leverages AI extensively for fraud detection. Their system analyzes user behavior and transaction patterns to identify suspicious activity, leading to a significantly lower fraud rate compared to the industry average.
HSBC:
This global bank utilizes AI to analyze vast amounts of data during the due diligence process for potential acquisitions. This allows them to identify potential risks and opportunities associated with the target company more effectively.
KKR:
This private equity firm leverages AI to analyze news articles, social media data, and other public sources during due diligence. This helps them gain a deeper understanding of target companies and potential risks before an investment.
FICO:
A leading provider of credit scoring and fraud detection solutions, FICO leverages AI in their suite of products to help financial institutions assess creditworthiness, detect fraud, and manage risk.
Challenges and Limitations in Fighting Frauds with AI
Data Quality and Bias:
AI algorithms require high-quality, comprehensive data for effective training. Ensuring data is accurate, unbiased, and representative of the target population is crucial for reliable decision-making.
Privacy and Security:
Financial data is inherently sensitive. Implementing AI systems necessitates robust security measures to safeguard this information and comply with stringent data privacy regulations.
Integration Complexity:
Integrating AI with existing financial infrastructure can be challenging due to compatibility issues between the new technology and legacy systems. Careful planning and adaptation are essential for seamless integration.
Explainability and Transparency:
Understanding how AI models arrive at their decisions can be difficult due to their complex nature. A lack of transparency can raise ethical concerns and hinder trust in the technology.
Addressing these challenges requires ongoing monitoring, continuous improvement, robust data security measures, proactive regulatory compliance efforts, and ethical considerations to ensure the responsible use of AI in finance.
Unlocking Potential: Generative AI in FinCrime
Looking ahead, Generative AI emerges as the next frontier in the evolution of AI for FinCrime programs. This branch of AI specializes in creating new data samples based on patterns discovered within training data. Financial institutions are exploring its applications in various areas, including:
Synthetic data generation:
Fabricating realistic yet anonymized data to train and test AI models for fraud detection.
Anomaly detection:
Identifying unusual patterns in financial transactions that might indicate potential fraudulent activity.
Data augmentation:
Artificially expanding existing datasets to address biases and improve the effectiveness of AI models.
While achieving accuracy in Generative AI presents challenges like securing high-quality training data and minimizing model bias, its potential to revolutionize FinCrime programs is undeniable. Generative AI paves the way for a future of more efficient risk management and robust compliance processes within the financial sector.
Revolutionizing Regulatory Risk Management: The Power of AI-Enabled RegTechs
RegTechs, driving efficiency and compliance in finance, are projected to reach a market value of $57.32 billion by 2030, offering advanced solutions to manage regulatory risks and enhance operational compliance. Institutions are increasingly turning to AI-enabled RegTechs to streamline compliance processes, ensuring trust in AI-powered financial systems and solid AI governance. These RegTechs address many constraints of traditional regulatory control systems, offering efficient solutions for managing risks and compliance. By automating critical procedures, supervising compliance communication, and delivering end-to-end visibility, AI-enabled RegTechs enhance operational compliance while ensuring standards and automating procedures critical for compliance management. Furthermore, they implement self-learning crisis management and provide adaptive ML and data analytics to anticipate compliance-related crises, reinforcing their pivotal role in enhancing regulatory risk management and operational compliance within the finance industry.
The financial industry is increasingly turning to RegTech solutions to meet regulatory requirements efficiently. AI-enabled RegTechs offer several advantages:
Regulatory Monitoring:
AI-enabled RegTechs help organizations keep pace with evolving compliance regulations by continuously monitoring and adapting to changes.
Cost Reduction:
By deploying AI, financial institutions can save costs associated with implementing and maintaining regulatory-compliant solutions.
Operational Efficiency:
AI-enabled RegTechs automate operations, delivering faster and more accurate insights compared to legacy systems.
Durability:
AI-enabled RegTechs enhance security by monitoring suspicious activities and rectifying incorrect decisions resulting from data manipulation.
Trade Surveillance:
AI analyzes trading data in real-time, detecting suspicious patterns and market abuse.
Fraud Prevention and AML:
AI-driven anomaly detection helps financial institutions detect and prevent money laundering and financial fraud.
In conclusion, the synergy between AI and big data marks a pivotal turning point in due diligence practices, particularly concerning financial fraud detection and risk management. As evidenced by reports from industry authorities such as FICO, PwC, and Experian, the efficacy of AI-powered fraud solutions is unmistakable. FICO's Fraud Trends Report 2021 underscores the remarkable potential, with machine learning models achieving a remarkable true positive rate of 90% or higher, substantially curtailing false positives. Meanwhile, PwC's Global Economic Crime and Fraud Survey 2023 and Experian's The State of Identity and Fraud 2023 illuminate how businesses worldwide harness AI and big data to fortify defenses against fraud, safeguarding both consumers and enterprises alike.
These reports serve as beacons, illuminating the path toward more robust fraud prevention measures and heightened regulatory compliance. The insights gleaned underscore the indispensable role of AI and big data in navigating the intricate contours of the modern financial landscape. With ongoing advancements in AI technology and data analytics, the future of due diligence holds promise for even more effective risk management strategies and heightened resilience against emerging threats. As financial institutions forge ahead, the symbiotic relationship between AI and financial diligence emerges as a linchpin in upholding the integrity, trust, and security essential for a resilient global financial ecosystem.
- #Due_Diligence
- #AI
- #Financial_Fraud_Detection
- #Risk_Management